The Boy Who Cried “Alert”
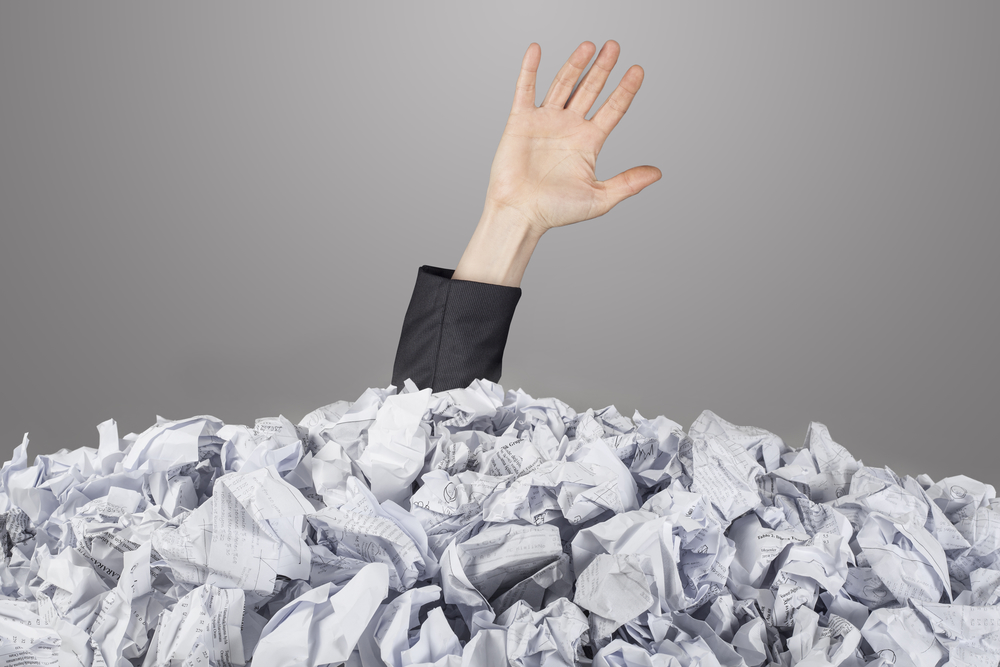
Later, he saw a REAL wolf prowling about his flock. Alarmed, he leaped to his feet and sang out as loudly as he could, “Wolf! Wolf!” But the villagers thought he was trying to fool them again, and so they didn’t come.
Amazing how little changes over 2000 years. Aesop captured the danger of false positives in “The Boy Who Cried Wolf,” and yet here enterprises and MSSPs are today, still dealing with the problem. Only today it’s not a mischievous little scamp playing tricks on the villagers. It’s their mischievous security infrastructures generating thousands of false-positive alerts, obscuring the smaller population of legitimate threats.
How do we solve the alert-overload problem? First, we have to stop living Einstein’s definition of insanity: doing the same thing over and over again and expecting a different result. Instead, we should follow the wisdom of Jerry Seinfeld in his classic “The Opposite” episode: “If every instinct you have is wrong, then the opposite would have to be right.”
Jerry has it exactly right. The way to slay the alert-overload beast is to change our approach from “opt-in” to the opposite: “opt-out.” In the traditional SIEM model, instead of setting security parameters that opt-in anomalous behavior for analysis, let’s do the opposite in a purpose-built platform and opt-out all the normal behavior. If you remove everything that is normal, you are left with only legitimate threats to investigate.
This makes perfect sense when you consider that “the abnormal” is wholly unpredictable. Previously unseen threats, combined with workplace trends that promote anomalous but innocent behavior − like mobile, inter-enterprise collaboration, telecommuting and globalization − have made it impossible to accurately define parameters for threats without also generating masses of false positives.
Now, let’s look at the opposite: the normal, which is predictable. Big data and machine learning make it possible to establish an accurate baseline of “normalcy,” which makes it possible to opt-out false positives before they enter the incident-response process. For example, if Susan is downloading files at midnight, opt-in systems will generate alerts because this is defined as abnormal behavior. The opt-out system, however, would know that this is normal behavior because Susan is a new mom working during off-hours, and would discard the false-positive alerts.
We live in a time where we can do amazing things with data. Unfortunately, technology often outpaces process, so we wind up with too much data and too little information. In security, this phenomenon manifests itself in the alert-overload problem. It’s time to end the broken process (opting-in suspicious behavior) and replace it with the opposite.
Too bad the Boy Who Cried Wolf didn’t think of this – he’d have more sheep in his flock.
By: Michael Lewis | Cybersecurity Engineer, CRITICALSTART
April 12, 2019
RESOURCE CATEGORIES
- Buyer's Guides(1)
- Consumer Education(39)
- Consumer Stories(2)
- Cybersecurity Consulting(4)
- Data Breaches(15)
- Data Privacy(43)
- Incident Response(2)
- Interview(51)
- MDR Services(59)
- MobileSOC(6)
- News(5)
- Press Release(101)
- Research Report(9)
- Security Assessments(2)
- Thought Leadership(19)
- Threat Hunting(3)
- Video(1)
- Vulnerability Disclosure(1)